Table of Contents
At the recent TechConnect Conference in the Washington, D.C., area,[1] it was evident that digitalization is driving all aspects of material research. Artificial intelligence (AI) and machine learning (ML) serve to inspire the discovery and design of new materials and the processes to manufacture them. Autonomous research and development (R&D) push the envelope for building predictive models and platforms that drive high-throughput innovation. How did we get here so fast, and where will this global megatrend take us?
In the Beginning…
In 2011, the Obama White House established the Materials Genome Initiative (MGI) for discovering, manufacturing, and deploying advanced materials twice as fast and at lower costs than traditional methods.[2] Today, the MGI is a federal multi-agency group that creates policy, resources, and infrastructure to support the U.S. in accelerating materials development. The interagency working group is led by the White House Office of Science & Technology and consists of all branches and programs of the Departments of Defense and Energy, the National Institute of Standards and Technology, NASA, the National Science Foundation, the Federal Drug Administration, the Networking and Information Technology Research and Development Program, and the U.S. Geological Survey.
Their strategic plan is to:
- Unify the materials innovation infrastructure, a framework of integrated advanced modeling, computational and experimental tools, and quantitative data
- Harness the power of materials data
- Educate, train, and connect the materials research and development workforce
- Ensure the U.S. maintains global leadership of emerging materials technologies in critical sectors including health, defense, and energy
In June 2024, the group conducted a workshop called Accelerated Materials Experimentation Enabled by the Autonomous Materials Infrastructure. The meeting had representatives from academia, industry, the national laboratories, and the interagency working group. The groups had working sessions on structural and functional metals, ceramics, soft matter, composites, and semiconductors.
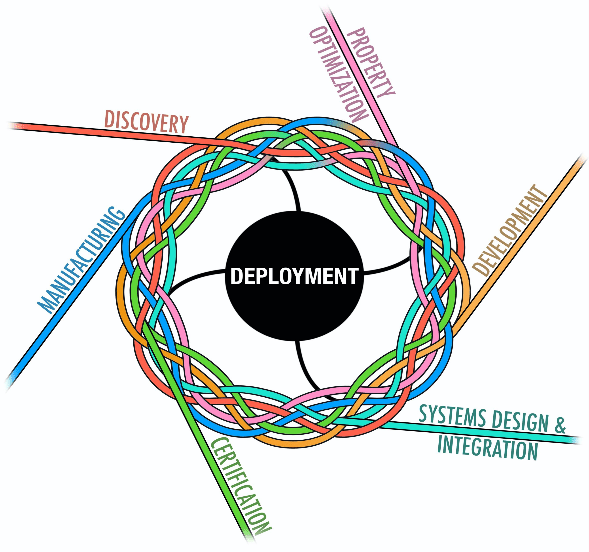
(Source: mgi.gov)
Key Elements of Material Design Using Autonomous Experimentation
Using AI to develop new materials has the potential to provide models of materials systems where none are currently available and to accelerate modeling where physics/chemistry models are well-understood. But there is a need for more data than is currently available, to lower costs and accelerate the rate of experiments.
The development of autonomous experimentation (AE) methods is the key to harnessing large amounts of materials data and rapidly designing new materials. The key steps to an AE workflow include:
- Artificial intelligence (AI), defined as a machine-based system that can, for a given set of human-defined objectives, make predictions, recommendations, or decisions influencing real or virtual environments.
- Machine learning (ML), a subset of AI associated with algorithms that enable systems to identify patterns and make decisions, and to learn through the acquisition of more data.
- Note: It is not uncommon for practitioners to use the terms AI and ML interchangeably.
- High-throughput screening methods allow for rapid, parallel testing of large numbers of materials.
- Computational models use physics/chemistry to predict the relationships between materials composition, microstructure, properties, and performance.
- Automation is a key element of AE, enabling robots and other machines to do repetitive tasks that would otherwise be performed by humans.
- Autonomy is the ability of machines to perform tasks and make decisions without constant human intervention. This authority does not absolve the human researcher of responsibility for actions by the AE system.
- Characterization is a broad term that captures methods for determining what material has been made, including compositional makeup. ML techniques have dramatically improved the ability to rapidly identify patterns, enabling faster characterization.
- FAIR is an acronym for findable, accessible, interoperable, and reusable data. It is a useful suite of concepts for determining whether a data resource and the workflows of an R&D operation allow collaborators to make maximum use of other data. Data is housed in data repositories, which can be assessed by the R&D community.
AE allows for asynchronous scheduling of work and continuous experimental campaigns, eliminating human working hours. The increase in speed of scientific experimentation not only allows more experiments to be performed but shifts from probing materials through individual experiments to enabling comprehensive mapping of the whole materials landscape. Additional benefits include lower labor costs and a drastic reduction in menial and repetitive tasks that can cause worker burnout.
Designing New Materials for Paints & Coatings
AI/ML and big data analytics will play a vital role in the paints and coatings industry as manufacturers must adopt digital transformation and invest in AI tools to remain competitive in the market. Market studies show AI-powered research in the chemical industry is growing at a CAGR of >30% and is predicted to reach more than $15 billion by 2033.[3] Companies already have vast amounts of institutional data generated over time, and AI/ML systems gather this data and curate it to form the bedrock of new AI workflows. Years of formulating by trial and error can be replaced with computer simulations that can predict performance and durability of new materials like resins and binders.
Many chemical raw material suppliers are already using these tools. For example, Dow reported at TechConnect that computational AI allows its teams to create products 80% faster, primarily because mundane tasks are done by robots. In addition, Dow has worked to build predictive toxicology and sustainability into its platforms.
Within coatings companies, AI/ML implementation can be expensive and require a lot of resources. As such, projects need to be chosen carefully to understand value creation. Hiring the best data scientists to work beside the research staff will help design experiments that solve high-value problems through the use of predictive modeling, data analytics, and process optimization. In the example shown here, the autonomous laboratory runs experiments in a continuous loop.[4]
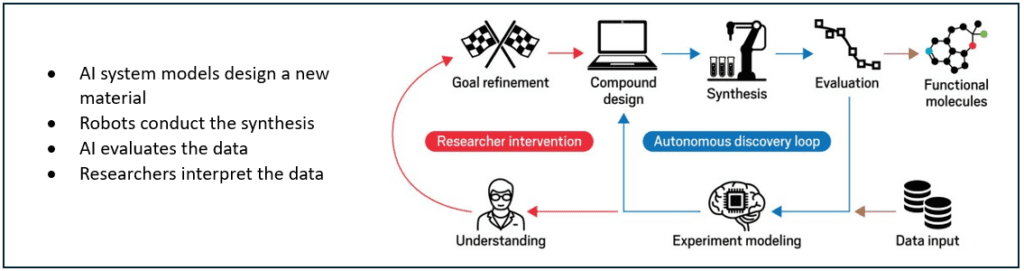
(Source: Conner Coley/MIT)
Once new materials are developed, AI/ML can provide formula optimization by identifying the best combinations of ingredients to give the desired results. Predictive modeling simulates how raw materials interact and provides the performance properties before anything is ever mixed in a laboratory. This reduces trial-and-error formulating by narrowing the field to the best, most likely candidates, allowing researchers to more quickly bring products to the market.
Other Contributions of AI/ML
The development of new materials is only one aspect of the tasks AI/ML can provide for paint and coatings companies. For example:
- AI systems can optimize equipment reliability using predictive maintenance programs that result in cost savings by identifying equipment failures before they occur, decreasing downtime and improving safety.
- AI-based quality control systems can ensure products meet consistent performance standards, accounting for variabilities in incoming raw materials and changes in the production process.
- Color matching has already been improved in some companies, as AI-based color matching systems have been developed to match colors with better accuracy, create new colors, or help customers choose the right colors for their needs.[5]
The Future is Now
The headlines are full of scary predictions about AI, and one may even hear echoes of movie theme music from The Terminator playing in the background. In reality, investments in programs like the MGI that coordinate with all the brightest minds in academia, industry, and the government serve to govern development and implement guardrails to ensure we are protected from the development of highly dangerous new materials.
AI did not happen overnight, but it is rapidly evolving. It represents a fundamental change in how we work. While paints and coatings represent a mature manufacturing industry, many businesses are investing in these new tools to innovate faster, create value for their customers, and provide a sustainable difference from the competition.
For more information, contact the author at vscarborough@chemquest.com.
Read in PCI.
References
1. “An AIGC-empowered Methodology to Product Color Matching Design,” Displays, January 2024, Volume 81.
2. TechConnect World Innovation Conference & Expo, June 17-19, 2024, Washington, D.C., www.techconnectworld.com/World2024/.
3. Materials Genome Initiative, www.mgi.gov.
4. “Artificial Intelligence in the Chemical Market,” Dimension Market Research, May 13, 2024.
5. “The Lab of the Future is Now,” C&EN, March 28, 2021, Volume 99, Issue 11.
How can we help you? Let’s start talking: https://chemquest.com/lets-start-talking/